Keyword [DeepTEN]
Zhang H, Xue J, Dana K. Deep ten: Texture encoding network[C]//Proceedings of the IEEE Conference on Computer Vision and Pattern Recognition. 2017: 708-717.
1. Overview
In this paper, it proposed Deep Texture Encoding Network (Deep-TEN) with novel Encoding Layer
- encoding layer. generalizes robust residual encoder

1.1. Contribution
- encoding layer. learnable residual encoding layer
- Deep-TEN. feature extraction, dictionary learning and encoding representation learn together
2. Methods
2.1. Residual Encoding Model


- X. visual descriptor
- C. learned codebook (learn K x D)
- r. residual vector
- E. output
S. smoothing factor (learned,
Concat K vectors and L2-norm.
- when K = 1, c = 0, it simplifies to sum pooling
- can deal with any input size
2.2. Deep-TEN

- Multi-size Training. 352x352, 320x320
- pretrained ResNet50
- K = 32
- weights of C and s randomly initialized with uniform distribution ±(1/√k)
3. Experiments
3.1. Multi-size vs Single-size training
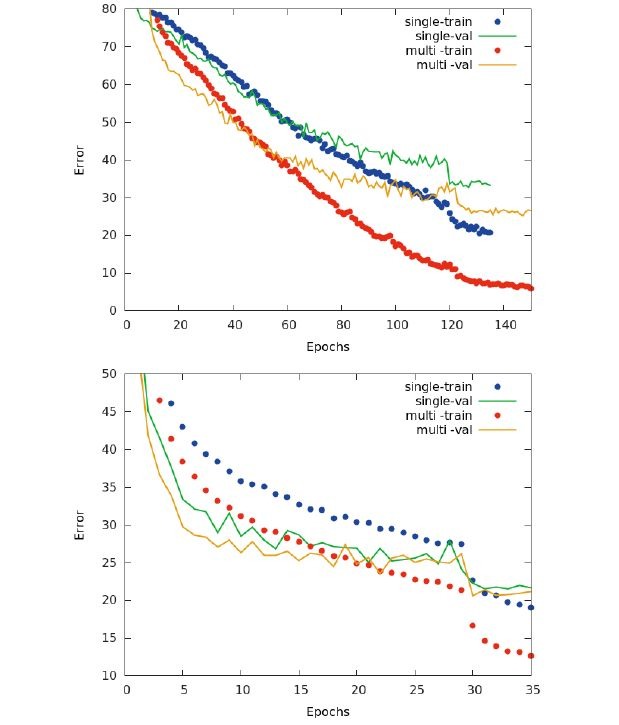
3.2. Comparison


